What does it take to build secure global infrastructure that trains algorithm models locally while ensuring data sovereignty and cultural appropriateness of AI applications?
Traditional cloud computing often involves latency, as data must travel back and forth between devices and centralized cloud servers. However, edge computing processes data closer to its source, significantly reducing latency. Gcore, a global infrastructure provider with cloud, edge computing, hosting, and security solutions, professes being able to offer this, thanks to its over 180 points of presence around the globe. In this region itself, Gcore has stood up 38 points of presence.
Artificial Intelligence (AI) has gained momentum in various sectors, from self-driving cars to customer service bots. For these applications to work efficiently, they need to process data swiftly and accurately. Gcore is a Luxembourg-based company that is responding to growing customer demand for enterprise-grade AI GPU cloud infrastructure. It observed that this demand is particularly for machine learning training on NVIDIA-powered servers and edge inference for AI applications.
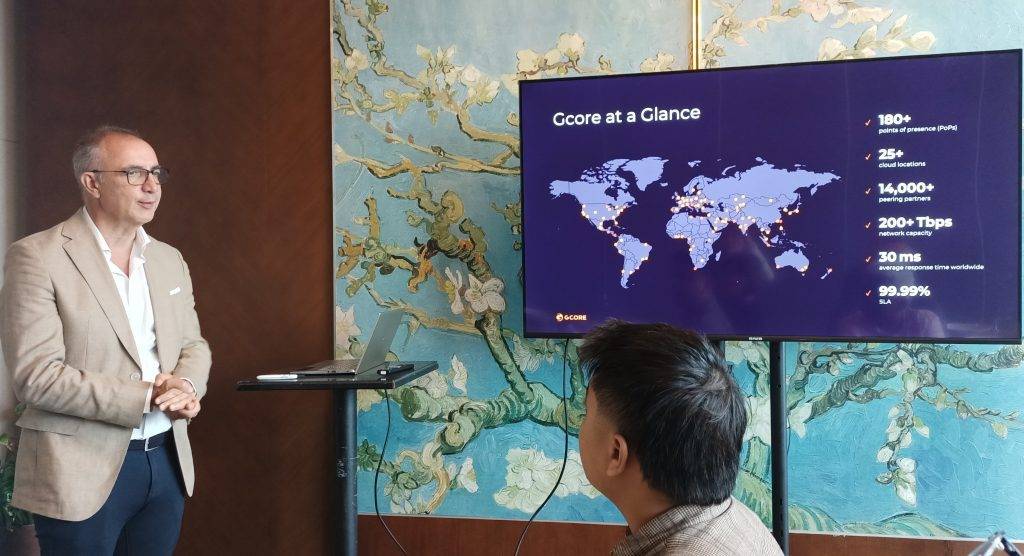
Fabrice Moizan during a media briefing to introduce Gcore.
When Gcore executives recently came to town to share about their expansion initiative to China, Japan, and South Korea, their ambitions were clear – to build secure infrastructure around the world, that trains AI models locally while ensuring data sovereignty and cultural appropriateness of AI applications.
“(When training GPT models) do I really want to feed (into) an algorithm which is based on the West Coast of the United States? It’s a cultural issue.” Chief Revenue Officer Fabrice Moizan said, giving the example of ChatGPT’s previous versions generating responses about American football when asked questions about soccer ‘football’.
“There is a need for countries, and even companies, to think about the security of data, and also sovereignty of their culture, using the right model.”
One example of how this can pan out is the Korean car manufacturer Gcore works with, to train its GPS voice guidance with data from Korea. The next stage for them is to bring this voice guidance capability over-the-air to the cars they have manufactured, wherever in the world they may be, by using Gcore’s global 180 points-of-presence (POP) infrastructure.
With POPs strategically located around the world, Gcore ensures that AI applications can utilize local compute resources. This significantly enhances response times and ensures that data remains within specific regions to comply with data sovereignty regulations.
Voracious demand for training and inference
As AI models grow exponentially more powerful and ubiquitous, they are driving unprecedented demand for specialized infrastructure. Gcore is positioning itself at the forefront of this shift, developing global and cloud-delivered GPU capabilities to enable better-performing AI applications, thanks to low-latency, improved reliability, cost-efficiency, and enhanced data privacy.
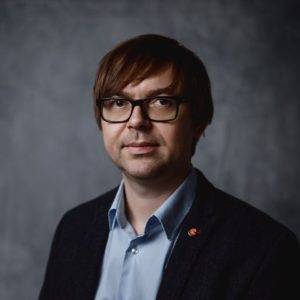
Andre Reitenbach, Gcore
It will also use edge caching and predictions to pre-load popular content at network edges for services like video streaming, which helps to reduce latency. In fact, Gcore founder, Andre Reitenbach said, “We preload data in local caches, bringing it as close as possible based on what the algorithms ‘predict’ our customers will do next.”
A lot of GPU resources go towards training AI models, using large amounts of data and compute power to develop accurate AI models. However, to be useful, these trained models then need to be deployed for inference – making predictions or classifications on new data in real-time – and Gcore noted there is a demand shift in resources to train models, to now offer inference services.
Gcore is leveraging this shift to inference with its global infrastructure to deploy trained AI models for real-time use cases like customer service, sales assistance, and content delivery. Specifically, they are partnering with telecommunications companies to provide GPU-powered AI inference services at the network edge with low latency. This allows trained models to be deployed and updated quickly for real-time applications.
Partnering on connectivity and infrastructure
The company is also actively partnering with telecommunications companies like Orange in Europe to help them deliver AI capability as a service. “It is great for us because many countries and companies do not have the money and the appetite to buy lots of GPUs,” Fabrice explained.
He noted that many telcos have legacy data centers needed for modern AI workloads. “They see hyperscalers like AWS eat their lunch because they have resources and are global. So, we need to partner to build AI “factories” and get more interconnection services around the world.
“Building cloud infrastructure is complicated, and legacy telcos have legacy data centers. AI is a new challenge for them because it requires real-time GPU services and data centers (to support it),” Fabrice said.
“It is great for us because many countries and companies do not have the money and the appetite to buy lots of GPUs.”
Gcore partners to deliver via the cloud, GPU compute capabilities in real-time that enable businesses to train AI models and offer features like smart GPS voice navigation, industrial automation, AI-powered conversational customer service, and more. AI demands not just advanced algorithms but also substantial computing power and efficient cooling systems.
Gcore addresses these needs with state-of-the-art data centers that utilize liquid cooling and other advanced techniques to maintain optimal performance and energy efficiency. Fabrice and Andre admitted that Gcore does not have any data centers, but will partner with companies around the world to offer AI-ready data center infrastructure.