Verta’s founder and now-Chief AI Architect at Cloudera, Manasi Vartak, sat down for a brief chat with CXpose.tech at the sidelines of Cloudera’s EVOLVE24 New York conference. It’s not everyday that one meets the creator of an AI-based startup from MIT, so the idea was to demystify AI as much as possible, while unravelling more about the problems Verta wanted to solve.
Verta, is an MIT startup that built software infrastructure for enterprise data science and machine learning teams to rapidly develop and deploy machine learning models.
Manasi Vartak founded Verta, based on her PhD thesis, a decade ago, wanting to solve a few key problems like model management, or version control for models. In an article, she was also vocal about needing to ensure the integrity of machine learning models as they become more prevalent in software and in our lives.
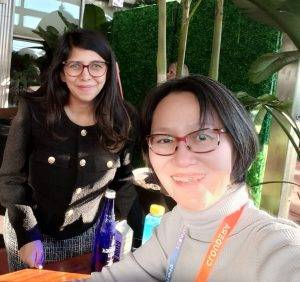
Manasi Vartak, Chief AI Architect with CXpose.tech’s Cat Yong
Manasi further explained, “You have one (version of a) model today, and you have another in a year, let’s say – so how do you make sure you are using the most up-to-date, secure versions, and then how do you put them into production? So, that’s what Verta specializes in.”
To give an idea of the quantum of models (and the ensuing messiness) involved, Manasi referred to Hugging Face, an open source repository, that has over a million models.
Operationalizing AI
“Operationalizing AI is kind of a unique word, so I’ll explain what it is,” Manasi said, adding that machine learning models are usually built in a research team consisting of data scientists and PhD holders.
But it does not stop there as the models need to be packaged, run, and integrated into software. “That’s the operationalizing part and that’s what we are focused on at Verta – taking models and managing, deploying, and then monitoring them.”
“You have one (version of a) model today, and you have another in a year, let’s say – so how do you make sure you are using the most up-to-date, secure versions, and then how do you put them into production? So, that’s what Verta specializes in.”
Monitoring the model quality involves looking at the kind of data being fed into it, and keeping track of whether the results the models produce have changed a lot In other words, “it’s to ensure that they are not making unpredictable sorts of judgements on the data,” Manasi said.
In such cases, model monitoring systems can detect performance degradation, signaling the need for retraining or adjustments.
The Chief AI architect pointed out, “We focus on the scaling of models (into production) and that’s very closely related to the AI inference announcement we have made.”
Helping machine learning models scale in production environments, aligns with Cloudera’s overall strategy to work with any model provider like Anthropic, Llama, and more, and on any platform be it a cloud infrastructure, or specialized hardware.
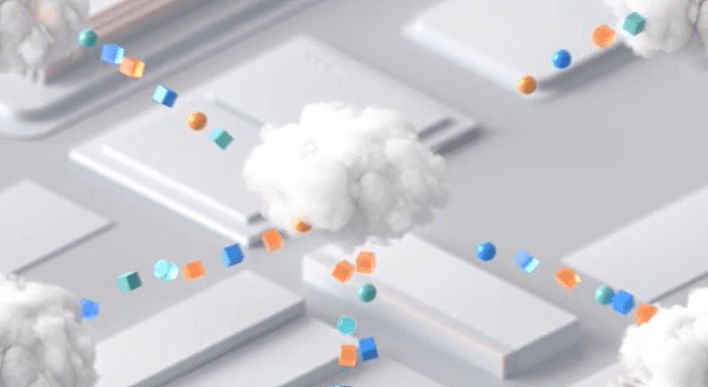
Pic courtesy of Cloudera.com
During his EVOLVE24 keynote, Cloudera CEO, Charles Sansbury, had talked a little about how the AI inference announcement Cloudera made with NVIDIA also allows this flexibility, underlining the latter company’s commitment “to provide its customers with the ability to take a best of breed approach and be successful with what they want to do with AI.”
Cloudera’s ability to to deploy models across private cloud, public cloud, and on-premise, is an advantage in enabling AI and agent-based use cases for their large enterprise customers.
Model trends, specialized models, and RAGs
Manasi highlighted a trend towards specialized AI models. “There’s unlikely to be one model that rules them all,” she observed. “What we’re going to find is that models become specialized for certain tasks.”
An example that she shared was Microsoft’s small language model (SLM), Phi-3 which is a very simple model trained on mainly coding tasks. “So, that’s where it is pretty good at and I expect there’s going to be a lot of models in the future, and enterprises can pick and choose the model that works for their use case,” said Manasi who is seeing use cases currently range from SQL assistants, all the way to customer support chatbots.
She observed that a lot of LLMs have been trained on publicly available data. When the LLM becomes really useful is when it is adapted to enterprise-specific data. And that’s why in the future, she sees enterprises will finetune public models to work in their particular and specific contexts.
”So, for example, the models that we might use on our legal contracts need to be fine-tuned to our way of writing legal contracts versus another organization’s way.”
When asked about RAG or retrieval augmented generation, Manasi described them as quite different from SLMs. While an SLM is a specific machine learning model, RAG is a technique to extend a model’s context by tapping into other sources of data.
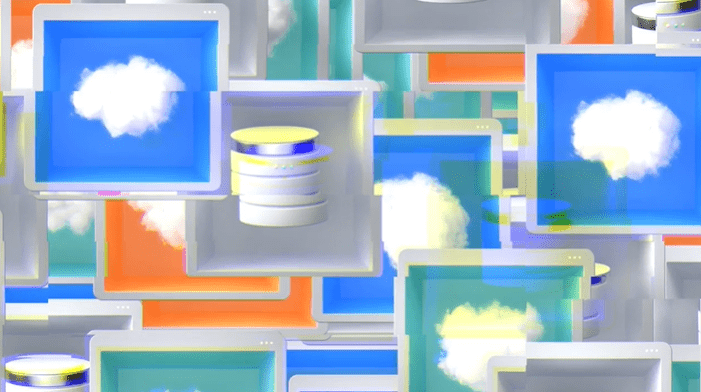
Pic courtesy of Cloudera.com
Reflecting on her experience with Verta and now at Cloudera, Manasi emphasized the importance of scale in operationalizing AI. “Cloudera serves enterprise customers at a much bigger scale,” she noted. “Now we can get all this great technology to 60% of the top companies in every vertical.”
The goals Manasi has set for herself in her newly minted role, align with the three pillars that are currently under her purview.
“So, are we helping more customers build AI themselves? That’s a metric reflected in our bookings and revenue. Within Cloudera itself, measurements can be of how sticky a product is, and the efficiency gains we are getting,” she concluded.
(This journalist was a guest of Cloudera’s to EVOLVE 24 New York)